Artificial Intelligence and Data Science Specialisation
Offline • 10 Months
Learn Artificial Intelligence and Data Science through our specialised program. Acquire practical skills and theoretical insights essential for success in this dynamic and high-demand industry.
No prior programming experience required
Scholarship available*
Placement assistance
About the course
Embark on a journey with our AI and Data Science Specialization course, a comprehensive online learning experience designed to equip learners with vital skills in artificial intelligence (AI), machine learning, and data science. This course offers hands-on experience with industry-standard tools and techniques, ensuring readiness to tackle real-world data challenges effectively. Whether you’re a novice looking to start a career in AI or a seasoned professional seeking to enhance your skills, this course offers the essential knowledge to excel in the dynamic fields of AI and data science.
- No prior programming experience required
- Industry graded projects
- Certificate of completion
- Placement assistance
- Guest lectures by industry professionals
- Learn by doing practical
Comprehensive curriculum
Introduction to Python Programming
- Introduction to the Jupyter Notebook environment. Basics Jupyter notebook Commands.
- Syntax, variables, and Data types.
- Data structures in python – List, Tuple, Set, Dictionary
- Conditional statements – if, Else, Elif.
- Loops – While & For Loops.
- Functions – lambda, recursions, map, filter & reduce functions.
- User-Defined Functions
- Errors and Exceptions- Try and Except
- List and Dictionary Comprehension
SQL
- Introduction to DBMS & RDBMS
- OLAP vs OLTP
- Database Design
- Database creation in MYSQL Workbench
- Single Table Queries – SELECT, WHERE, ORDER BY, Distinct, And, OR.
- Aggregation functions- Group by, max, min, sum,avg, etc.
- Multiple Table Queries: INNER, SELF, CROSS, and OUTER, Join, Left Join, Right Join, Full Join, Union, Union All.
- Subquery
- Analytics Function- Partition by, rank, dense rank, row number, lag, lead, etc.
Data analysis using python
- Pandas
- Numpy
- Data Cleaning – null and infinite values, outliers, capping, sanity
checks, data formatting, etc. - Types of Variables – Categorical & Continuous
- Visualization Libraries: Seaborn and Matplotlib
- Exploratory Data Analysis: Univariate, Bivariate, and Multivariate analysis.
Statistics
- Introduction to Statistics
- Basics of Probability
- Discrete Probability Distribution
- Continuous Probability Distribution
- Normal Distribution
- Poisson’s Distribution
- Bayes’ Theorem
- Central Limit Theorem
- Pearson Co-Relation, Co-Variance
- Creating confidence interval for the population parameter
- Characteristics of Z-distribution and T Distribution.
- Concepts of Hypothesis Testing: Null and Alternate Hypothesis
- Making a Decision and Critical Value Method
- p-Value Method and Types of Errors
- Two-Sample T-Test
- Two sample Z-test
- ANOVA, Chi-Square, A/B Testing
Supervised Learning – Regression
- Simple Linear Regression
- Multiple Linear Regression
- Ridge & Lasso Regression (L1 & L2)
Supervised Learning – Classification
- Logistic Regression
- KNN Algorithm
- Naive Bayes
- Decision Trees
- Random Forest
- Support Vector Machines
Supervised Learning – Ensemble
- Bagging and Boosting
- Xgboost Algorithm
- Adaboost Algorithm
- VotingClassifier
- Gradient Boosting Algorithm
Unsupervised Learning
- Introduction to Clustering
- K-Means Clustering: Linkage, Use of Elbow Curve & Silhouette
Score - Hierarchical Clustering
- Principal Component Analysis (PCA
- Introduction to Deep Learning and Neural Networks
- Introduction to Linear Algebra
- TensorFlow & Keras for Neural Networks
- Artificial neural Networks (ANN)
- Image Classification using Convolutional
- Neural Networks (CNN)
- Transfer Learning (LeNet 5, Alex Net, VGG 19 & 16, ResNet, Inception V3)
- Recurrent neural networks – Natural Language processing
- LSTMs – Long short-term memory
- GRUs – Gated Recurrent Unit
- LSTMs Applications- Language Modeling
MLOPS/Model Deployment
Machine Learning model deployment with Industry level architecture
- Data Transformation
- Data Analysis Expressions (DAX)
- Data Visualisation
- Connectivity modes
- Power BI report server
Learn how to take data from different sources (Extract), change it to the format you need (Transform), and put it into a database (Load).
Extract: Learn how to gather data from different sources like databases, spreadsheets, or online services.
Transform: Discover how to clean, format, and modify the data to fit your needs.
Load: Learn how to store the transformed data into a database or another storage system, making it ready for analysis and use.
- Industry relevant projects
- Mentorship by industry experts
- Resume building
- Mock interviews
Earn a certificate upon course completion
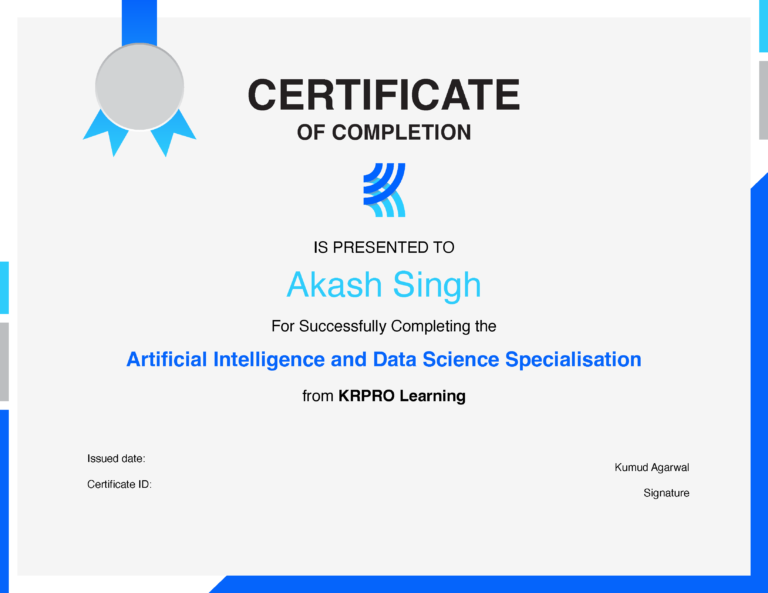
* Image for illustration only. Certificate subject to change.
The learning environment here is great. The coaching centre provides everything we need so that we are not disturbed while studying. The facilities in the classroom are top quality, making it perfect for learning. I believe it is worth it for anyone who wants to join. The faculty members are very supportive. Their teaching style is excellent and easy to understand. They always make sure we get the concepts and are always available to help us with any questions. We also have weekly doubt sessions which are very helpful. So far, I have really enjoyed studying at KRPro.
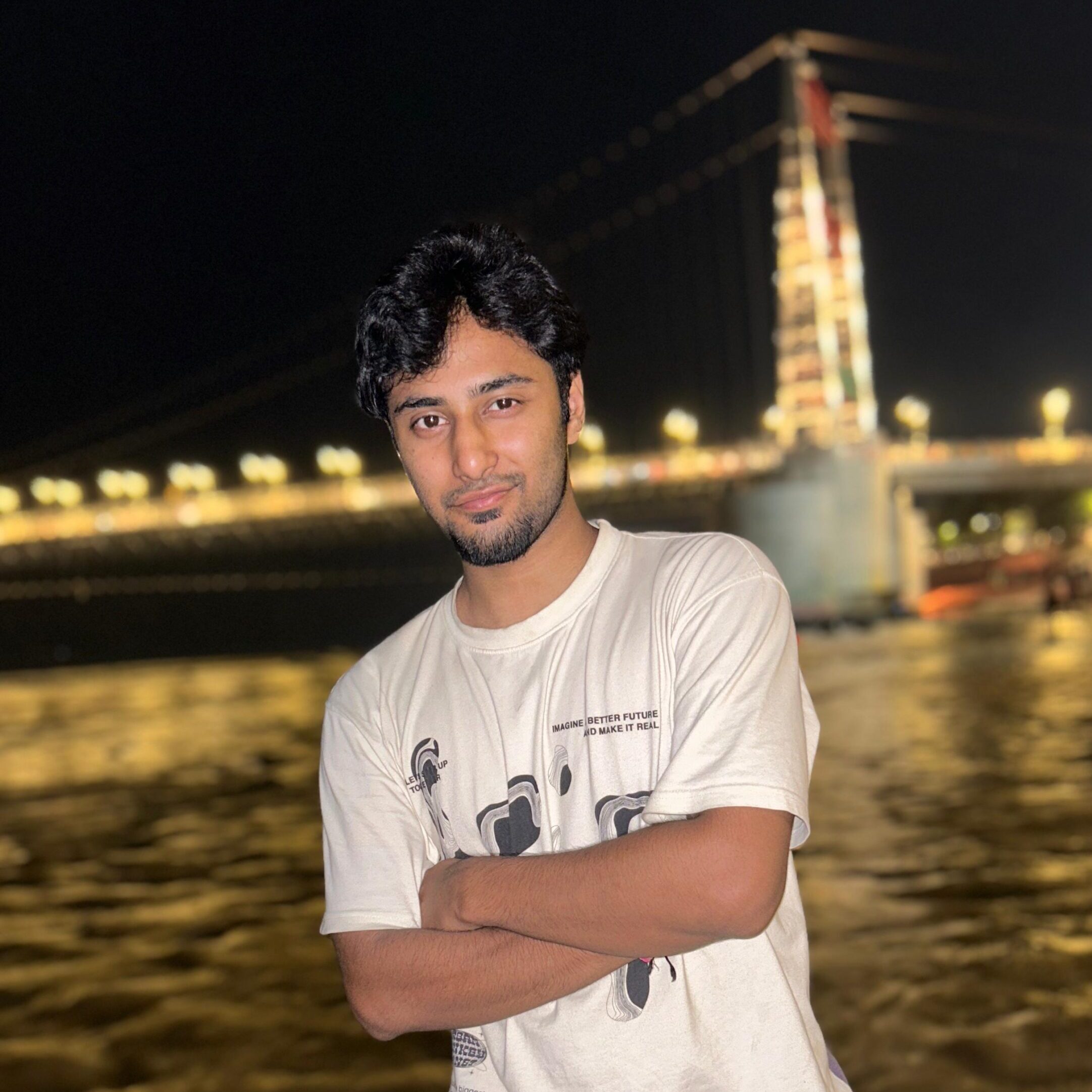
Upto 12 months of career support
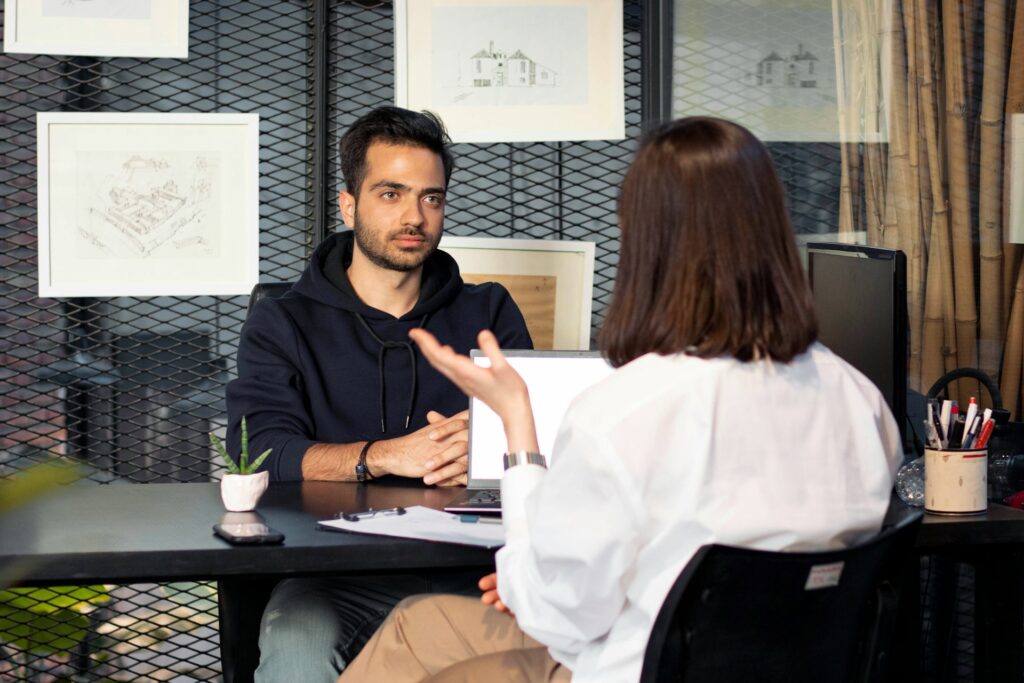
Mock interviews with industry experts
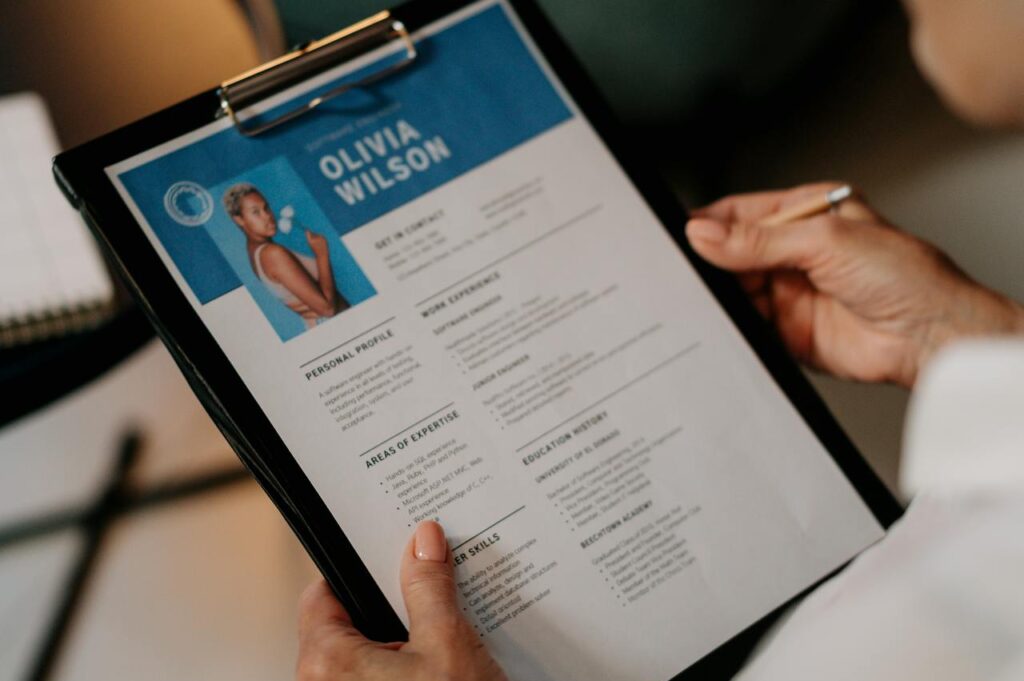
Resume building
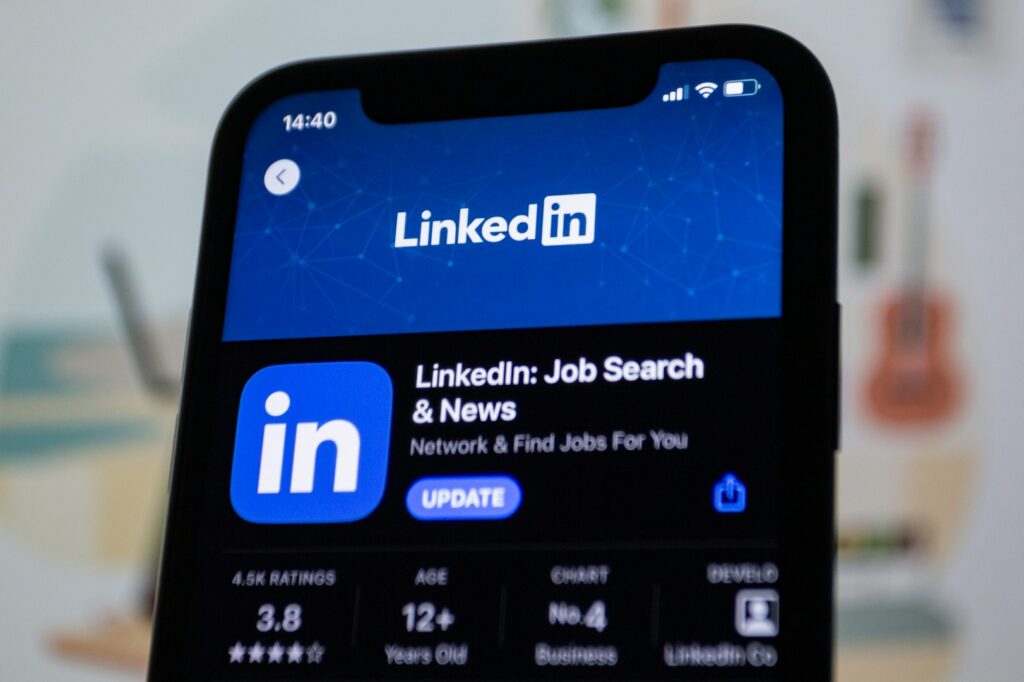
Naukri.com and Linkedin profile building
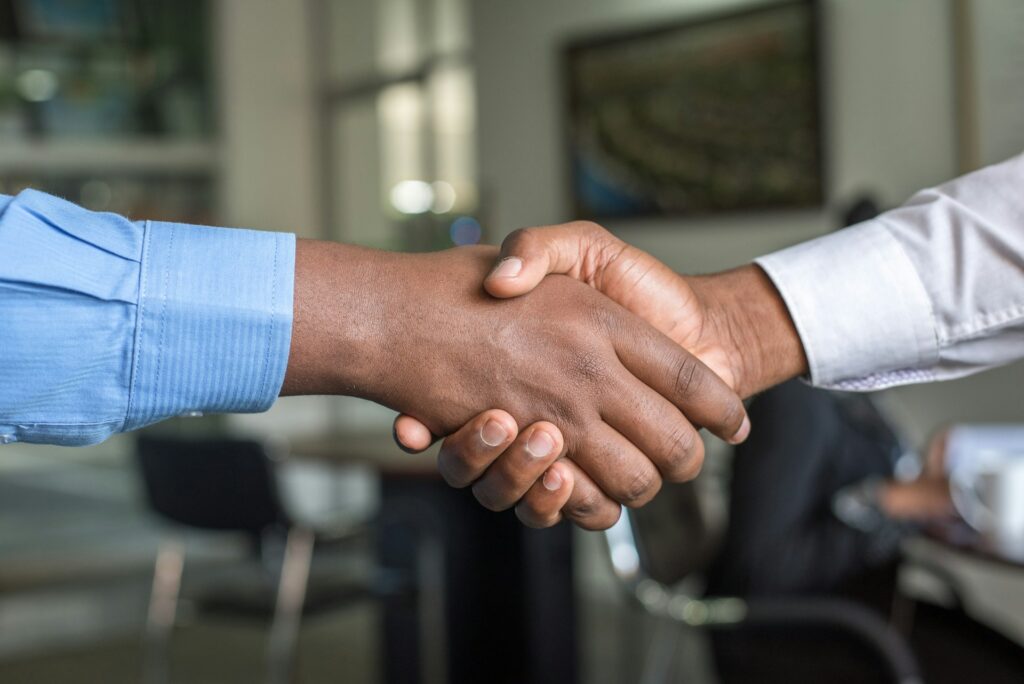
Placement assistance
Frequently asked question
This course is suitable for candidates pursuing graduation in any discipline or working professional. we will cover topics from beginner level to advance.
Fee structure can be divided into instalments. Moreover, we have loan partners which can help you in availing loan.
Yes, candidate have to use their own laptop.
There will be multiple projects for each learning module, although capstone project is the main project at the end of the course.
Candidates are evaluated in the course through a series of tests and quizzes.
Candidates are evaluated in the course through a series of tests and quizzes.